CrewAI Integration Guide
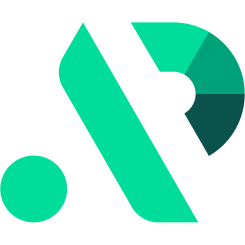
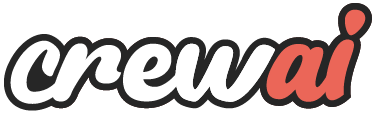
This guide will walk you through integrating CrewAI with APIpie, enabling you to build powerful multi-agent systems and orchestrate complex AI workflows with access to a wide range of language models.
What is CrewAI?
CrewAI is a fast and flexible open-source framework for orchestrating AI agents. It enables you to build autonomous, collaborative agent systems that can solve complex tasks through:
- Multi-Agent Orchestration: Create teams of specialized AI agents that collaborate to solve complex tasks
- Dual Paradigms: Use both autonomous Crews and controlled Flows for maximum flexibility
- Role-Based Collaboration: Each agent has a distinct role, goal, and backstory
- Process Management: Sequential, hierarchical, and custom execution flows
- Tool Integration: Equip agents with tools to interact with external systems
By connecting CrewAI with APIpie, you unlock access to a wide range of powerful language models while leveraging CrewAI's robust agent orchestration capabilities.
Integration Steps
1. Create an APIpie Account
- Register here: APIpie Registration
- Complete the sign-up process.
2. Add Credit
- Add Credit: APIpie Subscription
- Add credits to your account to enable API access.
3. Generate an API Key
- API Key Management: APIpie API Keys
- Create a new API key for use with CrewAI.
4. Install CrewAI
Install CrewAI and its tools using pip:
pip install crewai
# For additional tools (optional)
pip install 'crewai[tools]'
5. Configure CrewAI for APIpie
Set up your environment to use APIpie with CrewAI:
import os
from crewai import Agent, Task, Crew, LLM
# Set up with environment variables
os.environ["OPENAI_API_KEY"] = "your-apipie-api-key"
os.environ["OPENAI_API_BASE"] = "https://apipie.ai/v1"
# Or configure directly in your code
llm = LLM(
provider="openai",
api_key="your-apipie-api-key",
base_url="https://apipie.ai/v1",
model="gpt-4o-mini" # Or any model available on APIpie
)
Key Features
- Fast Performance: Lightweight, standalone framework built from scratch for speed
- Dual Paradigms:
- Crews: Teams of autonomous agents working together
- Flows: Precise, event-driven control for complex workflows
- Flexible Customization: Control both high-level workflows and fine-grained agent behaviors
- Tool Integration: Extend agents with powerful tools to interact with external services
- Process Management: Sequential, hierarchical, and custom execution processes
Example Workflows
Application Type | What CrewAI Helps You Build |
---|---|
Research Workflows | Multi-agent teams that research, analyze, and summarize topics |
Content Creation | Teams that plan, write, and refine documents and creative work |
Data Analysis | Collaborative analysis of financial data and market trends |
Business Process Automation | Complex multi-step workflows with business logic and tools |
Planning Systems | Agents that collaborate on planning trips, events, or projects |
Using CrewAI with APIpie
Basic Multi-Agent Research Crew
import os
from crewai import Agent, Task, Crew, LLM
# Configure APIpie as the LLM provider
os.environ["OPENAI_API_KEY"] = "your-apipie-api-key"
os.environ["OPENAI_API_BASE"] = "https://apipie.ai/v1"
# Alternatively, configure the LLM explicitly
llm = LLM(
provider="openai",
api_key="your-apipie-api-key",
base_url="https://apipie.ai/v1",
model="gpt-4o-mini" # Or any model available on APIpie
)
# Create a researcher agent
researcher = Agent(
role="Research Analyst",
goal="Find and summarize information about specific topics",
backstory="You are an experienced researcher with attention to detail",
llm=llm, # Use the configured LLM
verbose=True
)
# Create a writer agent
writer = Agent(
role="Content Writer",
goal="Create engaging and informative content from research",
backstory="You are a skilled writer who turns complex information into readable content",
llm=llm, # Use the configured LLM
verbose=True
)
# Create tasks for the agents
research_task = Task(
description="Conduct thorough research about artificial intelligence trends in 2025",
expected_output="A list with 10 bullet points of the most relevant AI trends in 2025",
agent=researcher
)
writing_task = Task(
description="Write a comprehensive article about AI trends in 2025 based on the research provided",
expected_output="A 1000-word article with clear sections covering each major trend",
agent=writer
)
# Create a crew with sequential process
crew = Crew(
agents=[researcher, writer],
tasks=[research_task, writing_task],
verbose=True
)
# Execute the crew
result = crew.kickoff()
print(result)
Adding Tools to Agents
import os
from crewai import Agent, Task, Crew, LLM
from crewai_tools import SerperDevTool # Search tool
# Configure your credentials
os.environ["OPENAI_API_KEY"] = "your-apipie-api-key"
os.environ["OPENAI_API_BASE"] = "https://apipie.ai/v1"
os.environ["SERPER_API_KEY"] = "your-serper-api-key" # For the search tool
# Create a researcher agent with search capabilities
researcher = Agent(
role="Market Researcher",
goal="Research market trends and competitor analysis",
backstory="You're a skilled market researcher with years of experience in competitor analysis",
tools=[SerperDevTool()], # Add the search tool
llm=LLM(
provider="openai",
api_key=os.environ["OPENAI_API_KEY"],
base_url=os.environ["OPENAI_API_BASE"],
model="gpt-4o"
),
verbose=True
)
# Create a financial analyst agent
analyst = Agent(
role="Financial Analyst",
goal="Analyze financial data and provide investment recommendations",
backstory="You're a financial expert who excels at interpreting market data",
llm=LLM(
provider="openai",
api_key=os.environ["OPENAI_API_KEY"],
base_url=os.environ["OPENAI_API_BASE"],
model="gpt-4o"
),
verbose=True
)
# Define tasks
research_task = Task(
description="Research the current state of AI chip manufacturing. Focus on major players, market share, and recent innovations.",
expected_output="A detailed report on the AI chip market landscape with key players and market trends",
agent=researcher
)
analysis_task = Task(
description="Based on the research, analyze the investment potential of top AI chip manufacturers. Consider growth prospects, risks, and competitive advantages.",
expected_output="An investment analysis report with recommendations for the top 3 AI chip manufacturers",
agent=analyst
)
# Create and execute the crew
crew = Crew(
agents=[researcher, analyst],
tasks=[research_task, analysis_task],
verbose=True
)
result = crew.kickoff()
print(result)
Using CrewAI Flows with APIpie
CrewAI Flows provide more precise control over execution paths:
import os
from crewai import Agent, Task, Crew, LLM
from crewai.flow.flow import Flow, listen, start, router
from pydantic import BaseModel
# Configure APIpie
os.environ["OPENAI_API_KEY"] = "your-apipie-api-key"
os.environ["OPENAI_API_BASE"] = "https://apipie.ai/v1"
# Define a state model for the flow
class ResearchState(BaseModel):
topic: str = ""
confidence: float = 0.0
findings: list = []
# Create a flow with structured control
class ResearchFlow(Flow[ResearchState]):
@start()
def initialize_research(self):
self.state.topic = "Machine Learning Applications in Healthcare"
return {"topic": self.state.topic}
@listen(initialize_research)
def conduct_research(self, inputs):
# Create a research agent
researcher = Agent(
role="Medical Research Specialist",
goal="Find and analyze the latest applications of ML in healthcare",
backstory="You're a specialist in healthcare technology research",
llm=LLM(
provider="openai",
api_key=os.environ["OPENAI_API_KEY"],
base_url=os.environ["OPENAI_API_BASE"],
model="gpt-4o-mini"
)
)
# Create a research task
research_task = Task(
description=f"Research the latest applications of machine learning in healthcare, focusing on {inputs['topic']}",
expected_output="A comprehensive report on ML applications in healthcare",
agent=researcher
)
# Create and execute a simple crew
research_crew = Crew(
agents=[researcher],
tasks=[research_task],
verbose=True
)
result = research_crew.kickoff()
# Update state with findings
self.state.findings.append(result)
self.state.confidence = 0.75
return result
@router(conduct_research)
def determine_next_step(self):
# Conditional routing based on confidence
if self.state.confidence > 0.7:
return "sufficient_data"
else:
return "need_more_data"
@listen("sufficient_data")
def generate_final_report(self):
# Create a report writing agent
writer = Agent(
role="Medical Content Writer",
goal="Transform research into readable reports",
backstory="You specialize in making complex medical technology understandable",
llm=LLM(
provider="openai",
api_key=os.environ["OPENAI_API_KEY"],
base_url=os.environ["OPENAI_API_BASE"],
model="gpt-4o-mini"
)
)
# Create a report writing task
report_task = Task(
description="Transform the research findings into a comprehensive report for healthcare professionals",
expected_output="A detailed report on ML applications in healthcare with recommendations",
agent=writer,
context="\n".join(self.state.findings)
)
# Create and execute the crew
report_crew = Crew(
agents=[writer],
tasks=[report_task],
verbose=True
)
return report_crew.kickoff()
@listen("need_more_data")
def request_additional_research(self):
# Logic for when more research is needed
return "More research needed to increase confidence in findings"
# Execute the flow
research_flow = ResearchFlow()
final_result = research_flow.start()
print(final_result)
Using CrewAI's YAML Configuration
CrewAI also supports defining agents and tasks using YAML files:
import os
from crewai import Agent, Task, Crew, LLM, Process
from crewai.project import CrewBase, agent, crew, task
from crewai_tools import SerperDevTool
from crewai.agents.agent_builder.base_agent import BaseAgent
from typing import List
# Configure APIpie
os.environ["OPENAI_API_KEY"] = "your-apipie-api-key"
os.environ["OPENAI_API_BASE"] = "https://apipie.ai/v1"
@CrewBase
class ResearchCrew():
"""Research crew for analyzing topics"""
agents: List[BaseAgent]
tasks: List[Task]
@agent
def researcher(self) -> Agent:
return Agent(
config=self.agents_config['researcher'],
verbose=True,
tools=[SerperDevTool()],
llm=LLM(
provider="openai",
api_key=os.environ["OPENAI_API_KEY"],
base_url=os.environ["OPENAI_API_BASE"],
model="gpt-4o-mini"
)
)
@agent
def analyst(self) -> Agent:
return Agent(
config=self.agents_config['analyst'],
verbose=True,
llm=LLM(
provider="openai",
api_key=os.environ["OPENAI_API_KEY"],
base_url=os.environ["OPENAI_API_BASE"],
model="gpt-4o-mini"
)
)
@task
def research_task(self) -> Task:
return Task(
config=self.tasks_config['research_task'],
)
@task
def analysis_task(self) -> Task:
return Task(
config=self.tasks_config['analysis_task'],
output_file='analysis.md'
)
@crew
def crew(self) -> Crew:
"""Creates the research crew"""
return Crew(
agents=self.agents,
tasks=self.tasks,
process=Process.sequential,
verbose=True,
)
# Execute the crew with inputs
inputs = {
'topic': 'Quantum Computing Applications'
}
result = ResearchCrew().crew().kickoff(inputs=inputs)
print(result)
Troubleshooting & FAQ
-
Which models are supported?
Any model available via APIpie's OpenAI-compatible endpoint can be used with CrewAI. -
How do I handle environment variables securely?
Store your API keys in environment variables or use a secure environment management tool. Never commit API keys to repositories. -
Can I use different models for different agents?
Yes, you can create different LLM objects for different agents, allowing you to optimize each agent for its specific task. -
How do I add custom tools to my agents?
CrewAI supports custom tools through the@tool
decorator or by creating a class that inherits fromBaseTool
. -
Can I mix Crews and Flows?
Yes, CrewAI is designed to let you use Crews within Flows for maximum flexibility, combining autonomous agent behavior with precise control flow. -
How can I save agent outputs to files?
Use theoutput_file
parameter when creating a Task to automatically save the agent's output to a file.
For more information, see the CrewAI documentation or the GitHub repository.
Support
If you encounter any issues during the integration process, please reach out on APIpie Discord for assistance.