Understanding CAG: AI's Conversation Memory
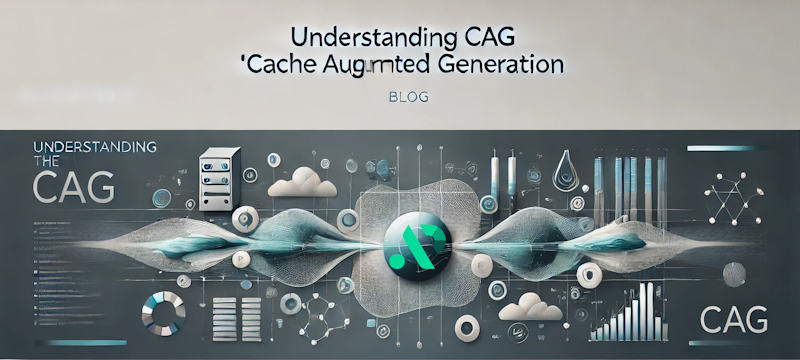
Ever noticed how your favorite AI assistant sometimes forgets what you were just talking about? Or how you need to keep reminding it of important context from earlier in your conversation? There's a solution that's changing the game: Cache Augmented Generation (CAG). Building on what we've learned about vector databases and RAG systems, CAG enhances AI responses by intelligently maintaining conversation context.
What is Cache Augmented Generation (CAG)?
Imagine if your AI could remember your entire conversation history and use that context to give you more relevant, personalized responses. That's essentially what Cache Augmented Generation (CAG) does!
Cache Augmented Generation is like giving your AI a working memory that:
- Maintains a history of your conversation
- Automatically includes relevant context from previous exchanges
- Helps the AI understand the full context of your current question
- Creates more coherent, contextually aware conversations
Unlike traditional AI interactions where each question is treated in isolation, CAG ensures the AI has access to your conversation history, creating a more natural and continuous dialogue experience.
Why CAG is a Game-Changer
The Problem CAG Solves
Let's face it - AI conversations can be frustrating when:
- Forgetful: The AI doesn't remember what you just discussed
- Repetitive: You have to keep providing the same context
- Disconnected: Each response feels isolated from the conversation flow
CAG tackles all these issues by maintaining conversation context across multiple interactions.
The "Aha!" Moment
Think about these common AI frustrations:
- "Why do I have to keep reminding it what we're talking about?"
- "I just told it that information two messages ago!"
- "It's like starting over with every question!"
CAG fixes these by:
- Automatically including relevant conversation history
- Maintaining context across multiple exchanges
- Creating a coherent, flowing conversation experience
How CAG Works Its Magic
Let's break down the process:
1. Conversation Memory: Beyond Single Exchanges
Traditional AI interactions treat each question in isolation. CAG is much smarter:
- Stores your conversation history in a structured way
- Organizes exchanges into meaningful sessions
- Maintains context across multiple interactions
- Uses vector similarity search to identify relevant past context
2. Context Augmentation: Enhancing Your Current Question
When you ask a new question:
- CAG analyzes what you're asking
- Identifies relevant context from your conversation history
- Augments your current question with this additional context
- Gives the AI model a more complete picture of what you're asking
This process is similar to how APIpie's Ragtune works with documents, but applied to conversation history instead.
3. Intelligent Response Generation: Better Answers
With the augmented context:
- The AI understands the full conversation flow
- Generates responses that acknowledge previous exchanges
- Creates more coherent, contextually relevant answers
- Delivers a more natural conversation experience
The result is what Google AI researchers call "conversational coherence" - the ability to maintain a consistent and natural dialogue over multiple turns.
CAG vs. Basic Prompt Caching: What's the Difference?
It's important to understand that CAG is different from simple prompt caching:
Basic Prompt Caching (OpenAI's Approach)
OpenAI offers a simple caching system that:
- Returns identical responses for identical prompts
- Primarily focuses on efficiency and reducing duplicate processing
- Doesn't enhance the context or understanding of the AI
- Works only with exactly matching inputs
It's like a simple lookup table - same input, same output.
True CAG Implementation (Anthropic's Approach)
Anthropic's approach to conversation memory is more sophisticated:
- Maintains conversation history across multiple exchanges
- Intelligently selects relevant context to include
- Enhances the AI's understanding of the current question
- Creates more coherent, flowing conversations
It's like having a conversation partner who actively remembers and references your previous exchanges.
Side-by-Side Comparison
Feature | Basic Prompt Cache | True CAG |
---|---|---|
Primary Purpose | Efficiency | Enhanced Context |
What It Does | Returns cached responses | Augments current question with context |
Conversation Awareness | None | High |
Implementation | Simple | More Complex |
User Experience | Faster responses | More coherent conversations |
Use Cases | Repeated identical queries | Natural flowing dialogues |
Real-World CAG Examples That'll Make You Say "Wow!"
Customer Support Magic
Before CAG:
Customer: "I have the premium plan."
AI: "Great! How can I help you with your premium plan today?"
Customer: "What features do I have access to?"
AI: "To tell you about available features, I'll need to know which plan you have."
After CAG:
Customer: "I have the premium plan."
AI: "Great! How can I help you with your premium plan today?"
Customer: "What features do I have access to?"
AI: "With your premium plan, you have access to advanced analytics, priority support, and unlimited storage..."
Personalized Assistance
- Remembers user preferences across multiple questions
- Maintains context about specific projects or tasks
- Creates a continuous, coherent conversation experience
Enhanced User Experience
Organizations implementing CAG have seen:
- Reduction in users having to repeat information
- Improvement in conversation coherence ratings
- More natural, human-like interaction patterns
CAG vs RAG: Short-Term Memory vs. Long-Term Knowledge
Both technologies enhance AI, but they serve fundamentally different cognitive functions:
The Human Memory Analogy
Think about how your own memory works:
-
Short-Term Memory (CAG/IMM): Remembers recent conversations and interactions. It's quick to access but limited in scope - like remembering what someone just told you a few minutes ago.
-
Long-Term Memory/Reference Library (RAG): Stores vast amounts of knowledge accumulated over time. It takes longer to access but contains much more information - like looking up facts in an encyclopedia.
CAG and RAG mirror these different memory systems:
Aspect | CAG/IMM (Short-Term Memory) | RAG (Long-Term Memory) |
---|---|---|
Primary Function | Remembers recent interactions | Accesses stored knowledge |
Information Source | Previous conversations | External documents/databases |
Access Speed | Extremely fast | Slightly slower (search required) |
Information Scope | Limited to past interactions | Vast knowledge repositories |
Primary Benefit | Speed & consistency | Accuracy & knowledge breadth |
Best Use Case | Repeated questions, conversation context | New information needs, research |
Working Together Like Human Memory
Just as humans use both short-term and long-term memory together, combining CAG and RAG creates a more complete AI cognitive system:
-
CAG/IMM provides the immediate context and conversation history - "What were we just talking about?"
-
RAG provides the factual knowledge and deeper information - "Let me look that up for you."
This combination creates AI systems that are both responsive and knowledgeable - they remember your conversation while also being able to retrieve specific facts from their "library" when needed.
APIpie's Integrated Model Memory (IMM): CAG Evolved
At APIpie.ai, we've taken CAG to the next level with our Integrated Model Memory (IMM) system. IMM is our advanced implementation of Cache Augmented Generation that offers unique capabilities not found in other solutions:
What Makes IMM Special
- Model-Independent Memory: Unlike traditional CAG systems tied to specific models, IMM works seamlessly across all supported AI models
- Cross-Model Context Retention: Start a conversation with GPT-4, continue with Claude, and switch to Mistral while maintaining complete context
- Multi-Session Support: Create independent memory instances for different users or applications
- Intelligent Expiration Handling: Configure custom expiration times for conversation contexts
How IMM Works
IMM leverages our Pinecone integration for efficient vector storage and similarity search, enabling:
- Automatic Context Management: No need to manually track conversation history
- Seamless Conversation Tracking: Maintain context across multiple interactions
- Smart Memory Controls: Configure expiration times and clear sessions when needed
- Effortless Implementation: Enable with a simple parameter in your API calls
Getting Started with IMM: Simpler Than You Think
Implementing our advanced CAG solution is surprisingly easy:
# Enable Integrated Model Memory for your API calls
curl -X POST 'https://apipie.ai/v1/chat/completions' \
-H 'Authorization: YOUR_API_KEY' \
-H 'Content-Type: application/json' \
--data '{
"messages": [{"role": "user", "content": "Your question here"}],
"model": "gpt-4",
"memory": 1,
"mem_session": "user123",
"mem_expire": 60
}'
Cross-Model Memory Example
One of IMM's most powerful features is maintaining context across different AI models:
# Start with GPT-4
curl -X POST 'https://apipie.ai/v1/chat/completions' \
-H 'Authorization: YOUR_API_KEY' \
-H 'Content-Type: application/json' \
--data '{
"memory": 1,
"mem_session": "cross_model_test",
"provider": "openai",
"model": "gpt-4o",
"messages": [{"role": "user", "content": "My favorite color is blue."}]
}'
# Continue with Claude, maintaining context
curl -X POST 'https://apipie.ai/v1/chat/completions' \
-H 'Authorization: YOUR_API_KEY' \
-H 'Content-Type: application/json' \
--data '{
"memory": 1,
"mem_session": "cross_model_test",
"provider": "anthropic",
"model": "claude-2",
"messages": [{"role": "user", "content": "What's my favorite color?"}]
}'
Learn more about implementing IMM in our comprehensive documentation.
CAG Best Practices: Do's and Don'ts
Do's:
- Create logical session groupings for different users or topics
- Implement appropriate session expiration times
- Combine with RAG for both context and knowledge
- Use consistent session IDs to maintain conversation continuity
- Structure conversations to build meaningful context
Don'ts:
- Don't mix unrelated conversations in the same session
- Don't set overly long session retention periods
- Don't rely solely on CAG for factual information (that's RAG's job)
- Don't overlook privacy considerations for stored conversations
- Don't neglect to clear sessions when conversations truly end
Frequently Asked Questions About CAG
When should I use CAG vs. basic prompt caching?
Use basic prompt caching when you're focused on efficiency for identical repeated queries. Choose CAG when you want to create coherent, contextually aware conversations where the AI remembers previous exchanges.
How does CAG improve conversation quality?
CAG dramatically improves conversation quality by maintaining context across multiple exchanges. This means the AI understands references to previous messages, remembers details you've shared, and creates a more natural, flowing dialogue.
Will CAG make my AI conversations more human-like?
Absolutely! One of the key differences between human and typical AI conversations is that humans remember what was just discussed. CAG gives your AI this same capability, making interactions feel much more natural and less repetitive.
Can I use CAG and RAG together?
They're perfect companions! RAG provides your AI with factual knowledge from documents and databases, while CAG gives it memory of the current conversation. Together, they create an AI that's both knowledgeable and contextually aware.
What infrastructure do I need for CAG?
True CAG requires vector storage capabilities and conversation management systems. With APIpie.ai's Integrated Model Memory, we handle all this complexity for you behind a simple API.
How does APIpie's IMM differ from other CAG implementations?
Our Integrated Model Memory is model-independent, allowing you to maintain conversation context across different AI models - a capability not found in other CAG solutions. This means you can switch between models mid-conversation without losing context.
The Future of CAG
The conversation memory landscape is evolving rapidly:
- More sophisticated context selection algorithms
- Multi-modal conversation memory (remembering images, audio, etc.)
- Personalized memory management based on user preferences
- Long-term relationship building between users and AI
- Integration with other AI enhancement techniques
According to recent research, conversation memory systems like CAG will become increasingly important as users expect more natural, coherent interactions with AI systems.
Ready to Supercharge Your AI Conversations?
CAG isn't just another tech buzzword—it's a practical solution that delivers real benefits:
- More coherent, flowing conversations
- Reduced need for users to repeat information
- More natural, human-like interactions
- Better user satisfaction and engagement
👉 Want to implement advanced conversation memory in your AI applications? Visit APIpie.ai and explore our Integrated Model Memory.
Join the growing community of businesses using APIpie's Integrated Model Memory to create AI experiences that truly remember what matters. The future of intelligent, contextually aware AI is here—are you ready to embrace it?